One of the responsibilities I had as the Director of Business Performance at Yotpo was to predict the amount of new business the sales department would generate each month. I got quite good at getting within only a few percentage points of the actual amount without needing to build complex models or machine learning.
In this post I'm going to show you my process and how you can start becoming a Nostradamus in your own organization.
Before we dive in it is important to mention that the goal of this post is to give you the tools to become as accurate as you can in predicting your company's sales numbers. In no way do I promise that my approach is perfect and even if you follow it you will still have months where your prediction will be off by a large margin. What I can promise is once you're done going through this post you will be smarter in how you look at your sales data and dramatically improve your prediction accuracy.
Step 1: Identify and calculate your business's leading indicators to new revenue
The first step in being able to predict your sales is to identify the leading indicates to your new revenue. I define leading indicators as factors which directly influence one or more specific KPIs. The amount of traffic and the visitor-to-customer conversion rate would be leading indicators to the new revenue KPI for an eCommerce business, as an example.
Some of the leading indicators for new revenue of a typical SaaS business include:
- Lead pipeline - The amount of leads available to the company's SDRs or account executives.
- Opportunity pipeline - The amount of revenue the company has in potential deals.
- Win Rate - A moving average of the company's ability to convert opportunities to deals. This rate can be dissected in many different ways as mentioned later in this post.
- Lead-to-demo conversion rate - Out of 100 leads generated, how many reach the demo stage?
You, as the head of data at your company want to know these numbers like the back of your hand. It is also important to monitor the changes in these metrics the best you can. To monitor the trends of complex conversion rates is very challenging without advanced BI tools and well structured data.
If you don't have a good idea of the values for the metrics I listed above then forget about predicting your revenues, your focus needs to be on better understanding your business.
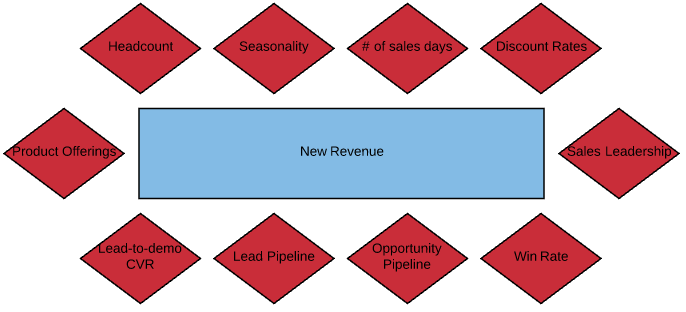
Step 2: Identify and calculate values for other influential variables
Now that you have identified and calculated the leading indicators, the next step is to write down values for other key variables.
You want to investigate each of the variables listed below and understand their influence.
- Number of sales reps on the floor ("headcount") - This is obvious but often taken for granted. You can get very granular here by looking at ramp up times, scheduled vacation time, sick days, new hires etc.
- Number of selling days - Each day that the team doesn't have to sell, especially towards the end of the month will have a huge impact.
- Discount rate - Has the team going overboard when it comes to discounts or perhaps the team isn't using discounts enough and the win rate is suffering?
- Product Offerings - Did your R&D just roll out an awesome update or new product which sales can leverage?
- Sales leadership - A major factor to the performance of your sales department is their leaders. You want to just verify that no major changes were made to both the personal and hierarchy of the sales management.
- Seasonality - Is it the beginning of a quarter or the end where departments are looking to spend their budget? Is it the high season for your industry, or are all the major decision makers in your niche on vacation?
- Marketing campaigns - Is your marketing department planning on launching a major campaign, or have plans to visit a popular conference?
Step 3: Identify positive and negative insights in your data
At this point you should have something like the information shown below:
Lead Pipeline
Total leads in pipeline: 154
Total leads in pipeline (new, as in pending first email / phone call): 35 (22% of pipeline)
Total leads pending demo (scheduled to have a demo): 15
# of account executives with under 30 leads: 3 (John, James, Maxine)
Opportunity Pipeline
Total opportunities in pipeline: 89
Total opportunities in pipeline in potential revenue: $1,345,950
Breakdown by stage and age:
- New: 23 ($347,829)
- 0 - 15 days: 15 ($226,845)
- 16 - 30 days: 8 ($120,984)
- > 31 days: 0
- Negotiating: 45 ($680,536)
- 0 - 15 days: 18 ($272,214)
- 16 - 30 days: 10 ($151,230)
- > 31 days: 17 ($257,092)
- Contract Sent: 21 ($317,585)
- 0 - 15 days: 21 ($317,585)
Win Rates
Opportunity to won rate (constraint of 90 days which is equivalent to 89% of sales): 27%
Contract Sent to Won rate: 76%
Negotiating to Won rate: 47%
Time to won: 89% of sales occur within 90 days, 75% of sales occur within 30 days
Lead-to-demo cvr
Flat at 8% with a constraint of 30 days.
Headcount & selling days
Selling days: 22 selling days
Headcount:
- 18 account executives on the books
- 15 reps working all 22 selling days
- 2 reps working 20 selling days
- 1 rep working 10 selling days
- (15*22) + (2*20) + (1*10) = 380 man-selling days (96% of full utilization)
Seasonality
This is an end of quarter month which are stronger months for the company's sales team.
Discount Rates
No major shifts in discounting trends.
Marketing Campaigns
The marketing department are planning the following:
- Launching a number of new pay-per-click campaigns
- End of quarter promotion via email to 50k leads / subscribers.
- Visiting medium-sized conference
Understand historical trends to determine impact
Once you've got values for all the different variables you want to look at historical trends that will help enrich your understanding of the data.
If you go through this entire process every month and are constantly looking at the data you will start to develop an almost 6th sense about what the data is telling you.
Some examples:
- A weak sales month often precedes a strong month because pipelines are replenished, certain deals which are taking longer close in the following month, and there is more pressure from the management.
- Seasonality is a big one. You need to understand which periods in the year are good for your business and which are not. Make sure you are analyzing things in terms of business quarters if you sell B2B.
- The performance of marketing has a direct impact on the size and quality of your pipelines. Keep an eye on Marketing's KPIs.
Step 4: Start putting it all together
The last step in the process is to put all the pieces of the puzzle together to come up with your prediction. At this stage you have all the variable information and a great understanding of how these factors influence sales so you can start building out your model.
Let's look at an example of how I would put all the information together to make a prediction.
Our example business has the following characteristics:
- The business closes between 400k - 500k dollars in new contractual value each month. This translates to roughly 19k - 23k per selling day. 300k was the worst month the company has had in the last 6 months, 500k was the best.
- The business has 10 account executives. No major changes in the last few months to the headcount and management structure. Moral is good.
- Last month product released a new add-on to the service which was highly requested by the market. VP of Product and VP of sales predict that it will help raise the average deal size by 20%.
- The lead pipeline is below average in volume and more than 60% of the pipeline is "old".
- The opportunity pipeline is healthy in terms of volume but 70% of it is considered at the pre-negotiation stage.
- This is a typical month. No seasonality to consider.
- The team has been discounting on average 70% of deals, this is up from around 65% of deals over the last 6 months.
- This month only has 19 business days because of a team event in the middle of the month and because the month starts on a Thursday.
- Lead-to-demo rate has been falling. Demo to opportunity cvr is flat.
- The marketing department are planning a number of campaigns to target leads which have expressed interest in getting the new product that was just released.
With the information above I'd predict the business will close $420k in new contractual revenue (22k per selling day). The way I get to this number is to focus on the most influential factors which are:
- Number of selling days
- Pipelines
- Marketing activities
- Product offerings
If you read through the list of variables again you will notice that most of the other variables are stable or cancel each other out.
Since the pipelines aren't in good standing, the new product offering is still fresh in the minds of the sales reps, and the number of selling days is lower than normal, I wouldn't expect this company to over deliver.
What would be interesting in our hypothetical scenario is how the company would perform in the preceding month. I would predict that it would have a very strong month because the pipelines would be better off, the sales reps have more experience selling the new product offering and marketing would have optimized their campaigns.
Other aspects not discussed
There are 3 additional aspects that I haven't covered yet in this post which may be relevant for your business.
The first is geography. If you happen to work for a global company with a distributed sales force then geography is very important, especially when looking at differences in seasonality, work culture and geopolitical climate. I see these factors as a separate layer that you will need to add to your metrics in order to take into account a global sales force.
The next factor is what the leadership of your company really cares about. In my example I focused on predicting contractual revenue, not actual revenue. To determine actual revenue from a specific cohort you will need to include collection rate, churn and a few other variables which complicates things. The reason I don't go that far is because most of these factors are stable and therefore you can skip going down that path. My goal is to measure the performance of the sales force and raise flags as soon as possible, not help determine budgets and other financial metrics, I leave that to the CFO and accountants.
The last thing I wanted to mention is that you should start doing these predictions 2 weeks before the end of the month. You will be able to take advantage of a lot more data and this will help improve your prediction accuracy. The negative side of this approach is 2 weeks before the end of the month might be too late to turn things around.
As you get better at understanding what factors are driving future results you can expand the distance between giving the prediction and the future you are predicting.